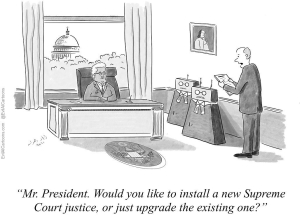
Predictive analytics, which can be understood as the application of artificial intelligence (machine learning and deep learning) based computer algorithms, to predict the future activities of a datasets based on their past or current behaviors are increasingly finding applications across different domains and sectors.
In the legal and civic fields, predictive analytics while previously known for its application in carrying out subservient tasks such as providing case law insights, mapping of contracts, vetting of legal provisions, are now being used for providing insights during legal trials and dispute resolution. These predictive jurisprudence softwares find their application in predicting recidivism probabilities of persons, investigating evidence, and even predicting the possible resolution of a civil dispute or a criminal charge based on the precedent and the legal infrastructure of the jurisdiction in which they operate.
In toto, predictive jurisprudence finds its application in three broad spheres, namely – (1) Predictive Justice: Criminal Sentencing, Settlement of Civil Disputes, Increasing Access to Justice; (2) Predictive Recidivism Software: Parole related decisions, Commutation of Criminal Sentences; (3) Legal Tools: Drafting tools, Contract Analysis Tools, and Legal Insight Tools.
The use of predictive jurisprudence and its development can be first found all the way back to the Supreme Court Forecasting Project (SCFP), a combined study conducted by students at the University of Pennsylvania, Washington University, and the University of California Berkeley, which was a statistics-based legal project that aimed to predict the outcome of every case argued in front of the United States Supreme Court in the year 2002. The backbone of this project was a statistics formula that when used, performed very well in predicting the decisions of the court to a degree of accuracy that even seasoned legal experts could not match. The statistics model predicted 75% of the court’s affirm/reverse results correctly, whereas the legal experts collectively predicted only 59.1% of the decisions correctly. Although the SCFP did not employ the use of computers per se, it provided proof of concept for the ideation that judicial decisions and the jurisprudence of courts are indeed parameters which can be readily predicted.
Since the SCFP, various companies and individuals have developed their digital products focused on assisting legal professionals by providing insights regarding legal materials or predicting the pattern of the judicial pronouncements, specificized based on the judges and courts across a plethora of legal matters such as settlement of insurance related claims, small cause matters such as traffic violations, granting of parole and commutation of sentences for convicts.
There are many companies who have well established digital products operating in the legal sphere, one of these is CaseCrunch, a UK based startup whose predictive jurisprudence application CaseCruncher Alpha showcased 86.6% accuracy in legal predictions by their algorithms, while the pool of 112 lawyers pitted against the CaseCruncher Alpha had an overall accuracy of 62.3%.
Another company flourishing in the predictive jurisprudence sphere is Loom Analytics which is a predictive analytics platform that features win/loss rates and judge ruling information but only for civil cases in select Canadian provinces, however, they are in the process of scaling up.
However, the established market powers in the predictive jurisprudence sphere are Prédictice– a French company in the business of providing legal case analysis (except for criminal cases). Another French company operating in this sphere is Case Law Analytics, which also works on providing legal analysis albeit much like Prédictice, it does not analyse criminal cases.
Another major player in the US market is Lex Machina which is owned by the global conglomerate LexisNexis and is in the business of providing legal analysis (including criminal cases) to legal professionals amongst other services such as insights such as how a judge behaves in a specific case, a compendium of crucial insights regarding the arguing styles, litigation experience which allows for persons to formulate an appropriate litigation strategy, Lex Machina also provides analysis of a party before a specific judge, courts or forums. Further, Lex Machina provides outcome-based analytics, timing-based analytics and helps in analysing the motions submitted to the court which helps professionals in crafting the appropriate motions to move the courts for specific causes.
Predictive jurisprudence is clearly a winner in terms of analysing not just volumes of data accurately but also identifying patterns in judicial behaviour which may not be visible to even the most seasoned experts.
The companies and private projects engaged in the use of predictive jurisprudence commercially, point towards an inherent market for predictive jurisprudence tools which have many users relying on the same to not only hone their professional skills and insights but also provide an increased access to justice across many jurisdictions. However, this brings us to our most important consideration yet- is it prudent to rely upon predictive jurisprudence software to carry out legal functions? And if so, what are the core tenants of designing and using such software.
The use of AI in this context relies on two specific considerations- the domain or sector in which it will operate and the characteristics of the tasks it will carry out. For example: the AI based software when applied to the legal sector if carries out administrative tasks such as retrieval or organisation of files, can be considered a low-risk AI and therefore, its users need not be made to go through a wide array of disclosure and notifications that they are interacting with an AI system. However, in the case where the AI based software is carrying out complex tasks such as legal deliberation, which would normally require a degree of expertise, the AI will be classified as a high-risk AI since any mistakes or shortcomings can have a direct impact on the life and liberty of an individual.
This brings us to our next crucial consideration about what core tenets are supposed to be kept in mind while designing a predictive jurisprudence-based AI software. First and foremost, a strict compliance with data protection laws takes centrestage in such a software, making a wonderful case for incorporating privacy by design.
Secondly, all legal procedures across jurisdictions- whether civil law, common law etc., have a common abidance to the principles of natural justice which are namely- (1) Adequate Notice; (2) No presence of Bias and; (3) Providing a reasoned order for all delibertations.
This brings us to an important component in all predictive jurisprudence-based AI applications- a degree of explainability. Explainable AI (XAI) has made many developments in the recent times, and a degree of explainability in a predictive jurisprudence application is crucial in as much as it allows for natural persons to readily rely on them since they understand the reasoning behind the computational results of the AI. The use of XAI as a core design tenet will also enable the predictive jurisprudence application to function independently in low-risk or moderate-risk tasks.
In their current form, since most predictive jurisprudence are far from perfect, they require human oversight and thus function to accentuate the legal analysis of lawyers, judges, and other legal professionals.
The EU Agency for Fundamental Rights (FRA) published a report (The European Union Agency on Fundamental Rights, 2020) in 2020 under the directorship of Michael O’Flaherty titled “Getting the future right: AI and fundamental rights” (FRA Report).
The FRA Report mentions the requirement for adequate disclosure while using the AI-based predictive jurisprudence technologies, this will provide the persons with the successful opportunity to complain about the use of AI and challenge the decisions which have been arrived upon based on the AI as this grievance and complaints mechanism is crucial for upholding the access to justice. The following are crucial to be reported to persons using the predictive jurisprudence based tools, in order to ensure access to justice-
- Making people aware that AI is used
- Making people aware of how and where to complain (A proper and designated grievance redressal mechanism)
- Making sure that the AI system and decisions based on AI can be explained (Use of XAI)
Many legal scholars have voiced their concerns about the use of predictive jurisprudence by courts and legal officers asserting that justice must be deliberated and not predicted hinting at the possibility of its users succumbing to automation bias. This concern has been adequately addressed in the current scenario as currently and until the time the AI based predictive jurisprudence software cannot explain the reasoning behind its computational results, it will operate only under the supervision of a natural person who may use the results as a component to deliberate upon while arriving at their well-reasoned decisions, while primarily relying on their own experiences and expertise.